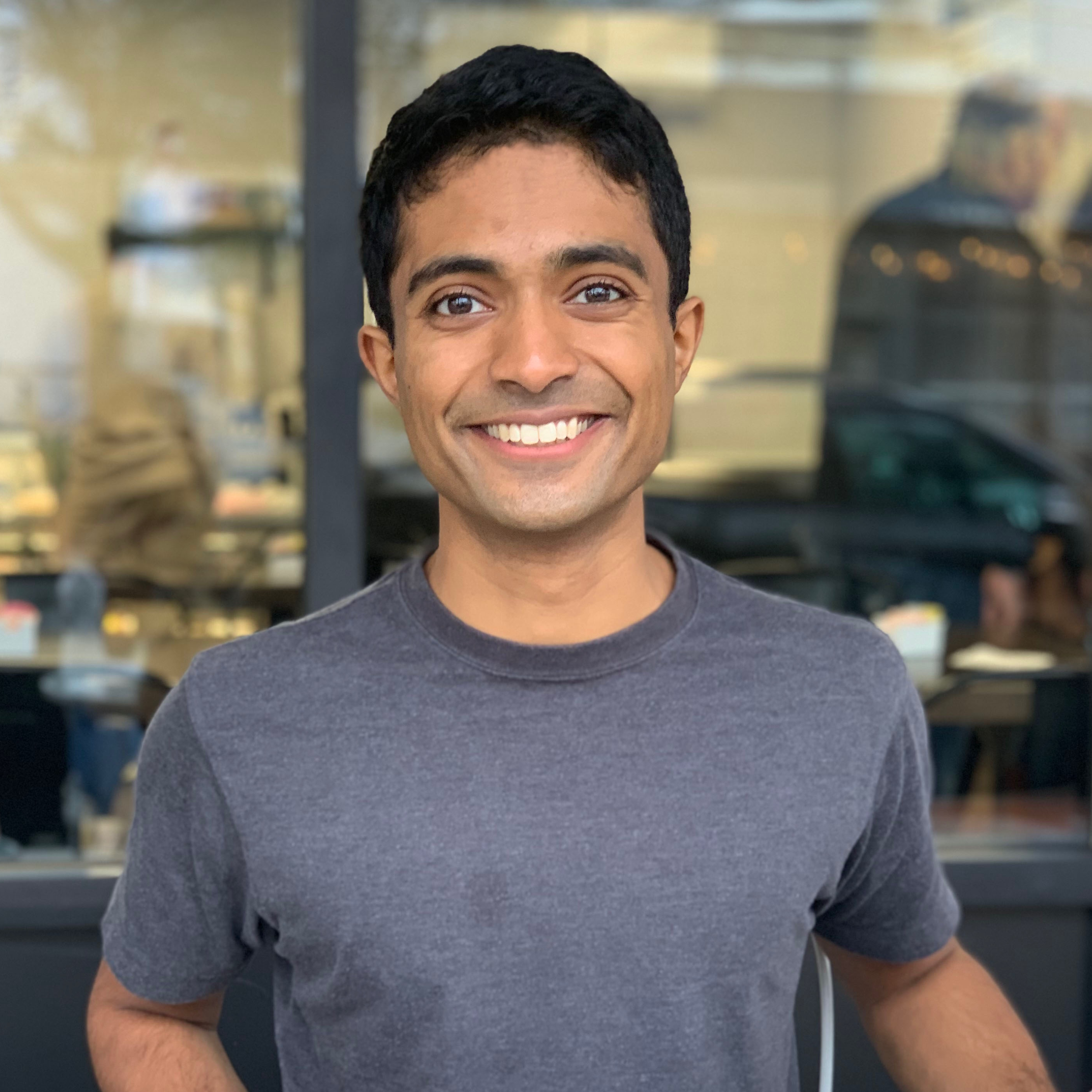
PhD Candidate • Harvard-MIT Health Sciences and Technology
Group: Computer Science and Artificial Intelligence Laboratory
Advisor: Dr. Polina Golland
I am a PhD student at the Harvard-MIT Program in Health Sciences and Technology, advised by Polina Golland. My research focuses on developing computer vision models to extract crucial 3D information from 2D medical imaging, aiming to advance diagnostics, image-guided interventions, and surgical robotics.
Research
Differentiable Rendering + Minimally Invasive Surgery
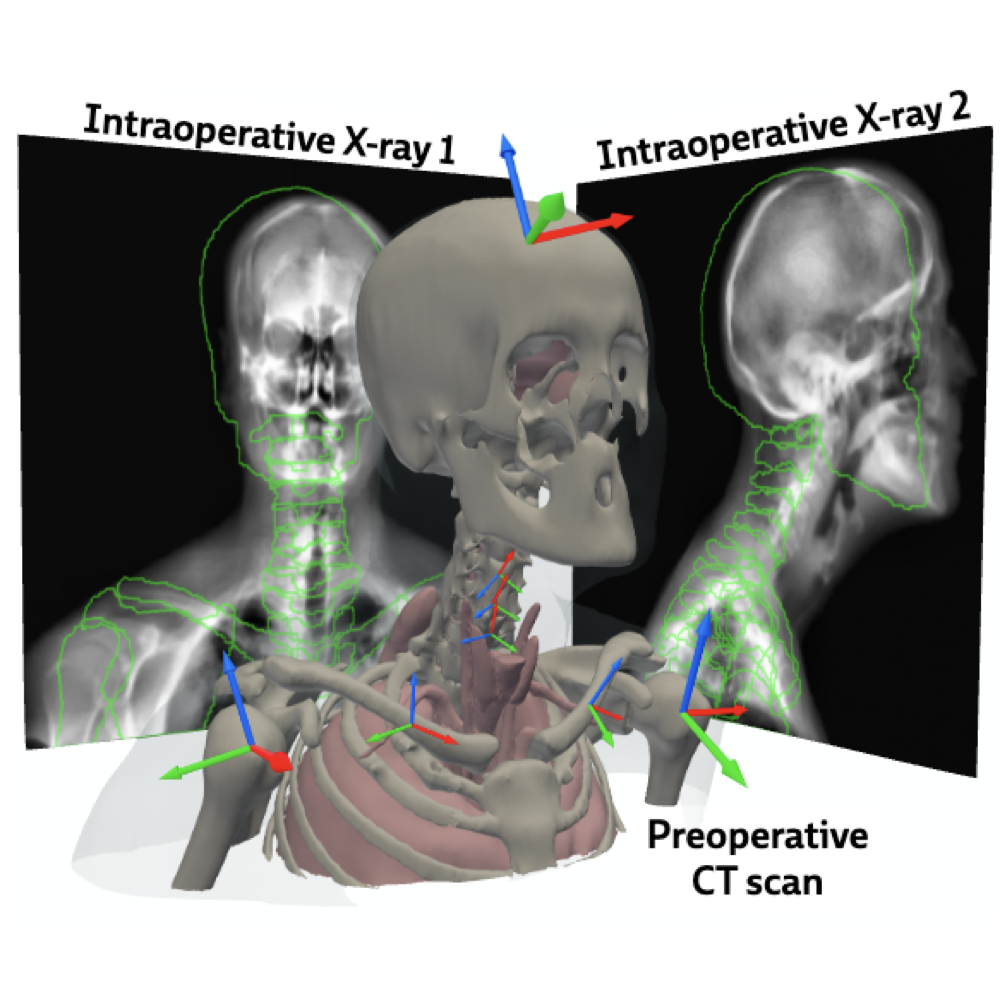
PolyPose: Localizing Deformable Anatomy in 3D from Sparse 2D X-ray Images using Polyrigid Transforms
arXiv, 2025
PolyPose
is a fully deformable 2D/3D registration framework that accurately solves highly ill-constrained sparse-view and limited-angle registration with polyrigid transforms.
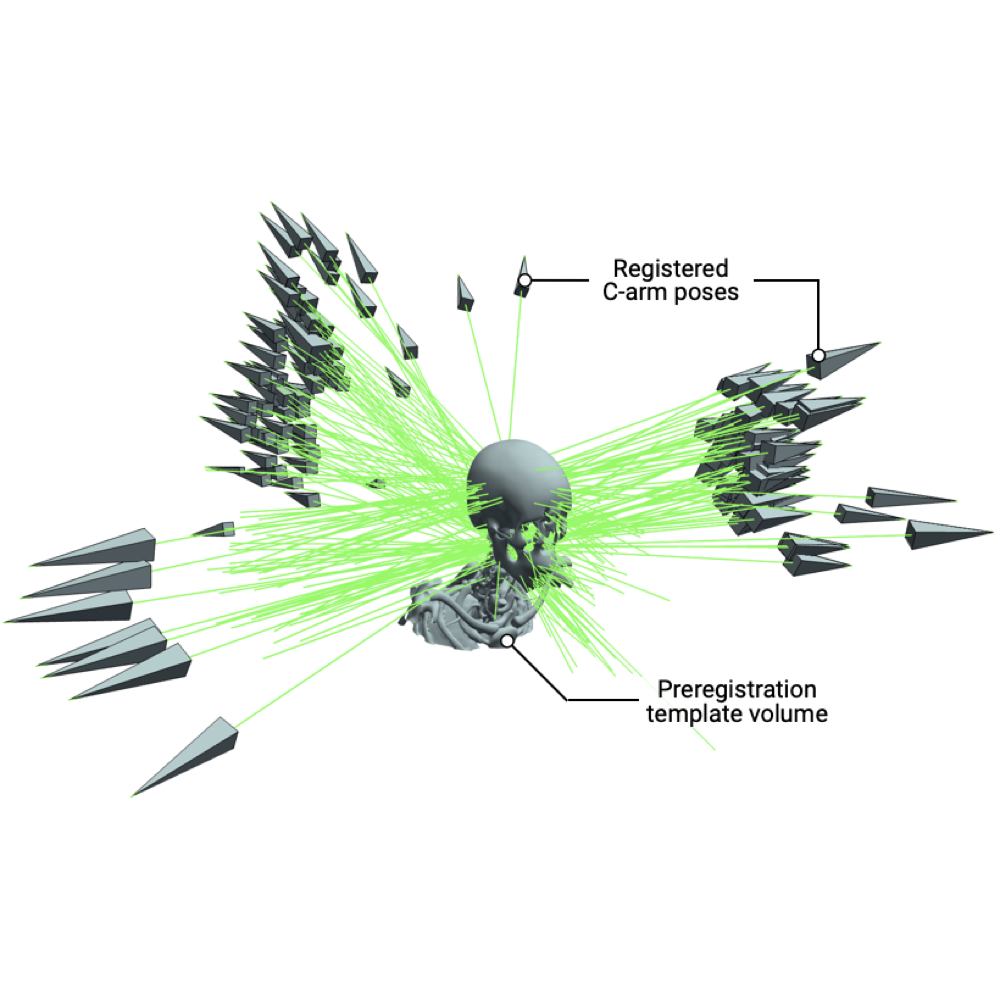
Rapid Patient-Specific Neural Networks for Intraoperative X-ray to Volume Registration
arXiv, 2025
We present xvr
, a CLI/API for training patient-specific 2D/3D registration in models in 5 minutes (100x faster than DiffPose
).
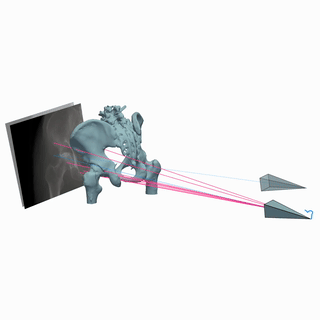
Intraoperative 2D/3D Image Registration via Differentiable X-ray Rendering
CVPR, 2024
We use X-ray renderering to develop DiffPose
, a self-supervised framework for differentiable 2D/3D image registration that achieves sub-millimeter registration accuracy.
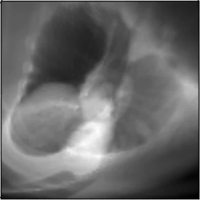
MICCAI Clinical Image-based Procedures Workshop, 2022
We present DiffDRR
, a differentiable X-ray renderer that can be used to solve inverse problems in X-ray imaging with deep learning (e.g., registration or reconstruction).
Drug Discovery
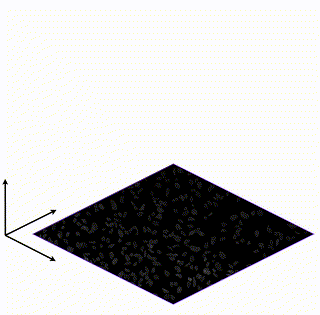
Learning Interpretable Single-Cell Morphological Profiles from 3D Cell Painting Images
CVPRW, 2024
Society of Biomolecular Imaging and Informatics, 2023 (President's Innovation Award)
Supervised deep learning models are used ubiquitously throughout image-based drug discovery. We discover a mechanism by which these models cheat and propose an interpretability metric to quantify the level of confounding.
Statistical Graph Theory
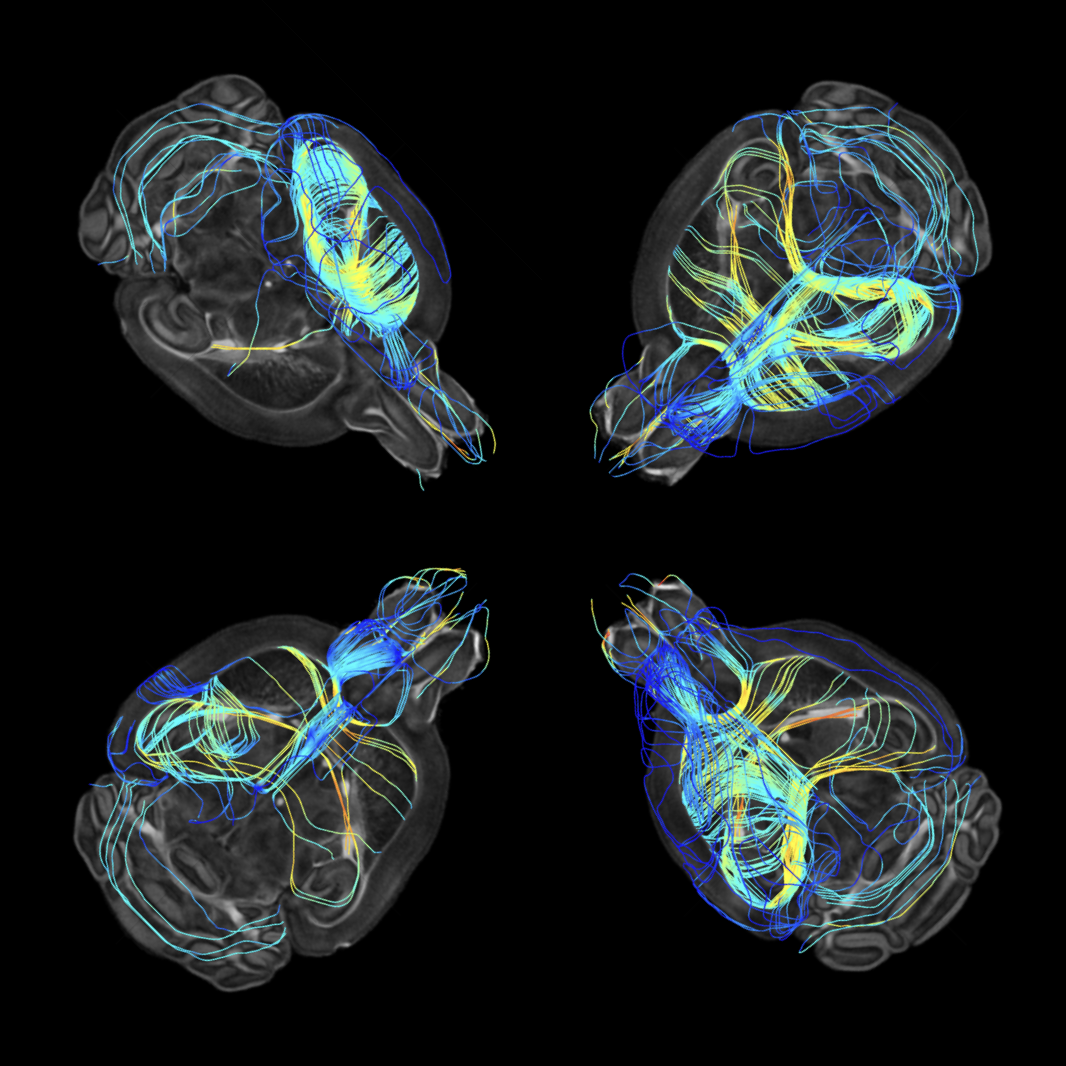
Multiscale Comparative Connectomics
Imaging Neuroscience, 2025
We introduce a set of multiscale hypothesis tests to facilitate the robust and reproducible discovery of hierarchical brain structures that vary in relation with phenotypic profiles.
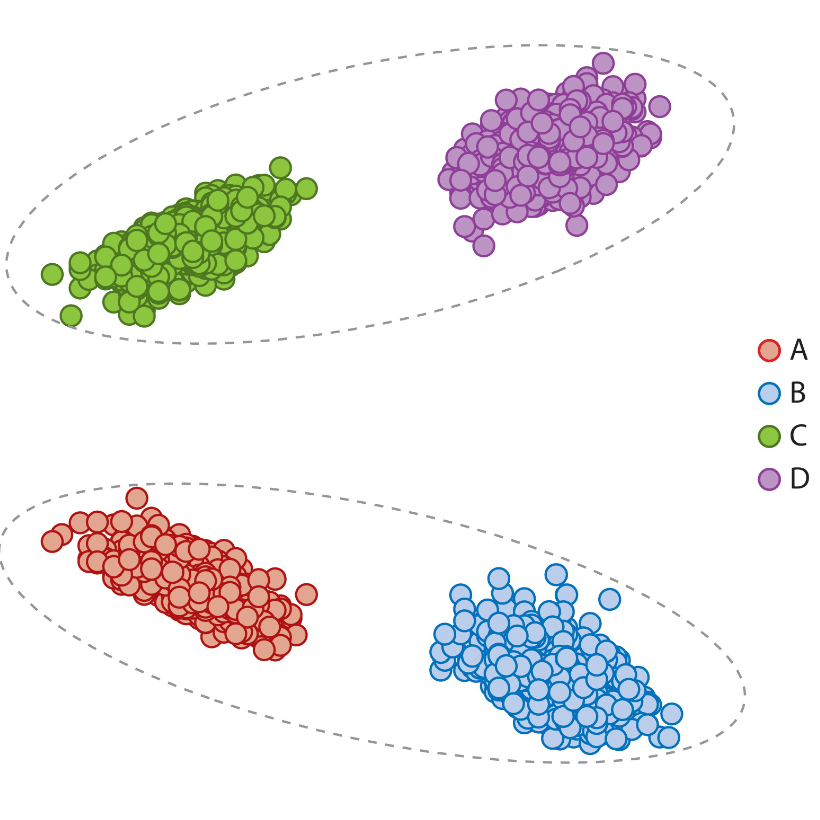
Annual Review of Statistics and Its Application, 2021
From a statistical graph theory perspective, we review models, assumptions, problems, and applications that are theoretically and empirically justified for analysis of connectome data.